Real Estate Meets AI
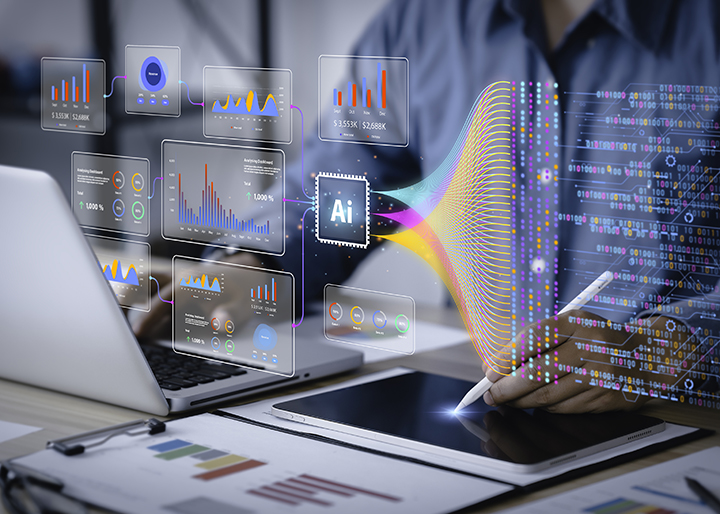
Productivity Gains and Persistent Limitations
by Jonas Bordo
In the fall of 2022, OpenAI released ChatGPT and made the power and promise of artificial intelligence (AI) a tangible reality. To date, the impact has been most profound in the area of human productivity.
For example, here at Dwellsy, we precisely map every SFR and apartment address that comes into our system (hundreds of thousands per month), and what was once a labor-intensive process is dramatically faster and more accurate with help from AI. Code debugging — one of the most painstaking and unpleasant tasks for our developers — is now (usually) a breeze. Processing emails is dramatically faster with AI reviews and drafts. It is almost as if we have doubled our team size — for only $20 per month per team member in most cases.
What AI makes possible with scaled data is nothing short of miraculous. A human can tell in a heartbeat whether or not one single-family home has a garage. But, to look at tens of thousands of them each day and do the same? There is no one human capable of or willing to deliver at that scale, but Large Language Models (LLMs) are happy to take on the task — and they can handle it in minutes and without complaint.
LLMs have had a similarly profound impact on our analytical ability. We can feed financial statements into LLMs like ChatGPT, Claude or Gemini and query the financials to get insights in seconds. We can give the LLM an enormous data set and ask for any kind of analysis. They are not always right (like the humans they are built to emulate), but they make an invaluable thought partner in our work.
While AI is becoming indispensable, it has serious limitations that are not going away anytime soon. Let’s break them down.
Without Good Data, AI is Pointless (or Worse)
AI is only as good as the data it uses — the challenge of “garbage in, garbage out” has not changed. Poor-quality data, incomplete datasets, or outdated information can lead to inaccurate predictions and flawed decisions. And SFR is rife with data challenges.
Here are some common issues that AI runs into:
» Offline Properties // In SFR, many properties exist solely offline — rented via a yard sign and managed in someone’s notebook, or an Excel sheet. As a result, AI will miss many reference properties that could be invaluable for analysis.
» Data Fragmentation // Even when digitized, many owners and operators do so on in-house platforms that are not shared, so much of the data is behind enterprise firewalls and inaccessible to AI.
» Old Data // SFR evolves rapidly due to factors like new developments, economic shifts, or regulatory changes. Too much data is historical and AI models may rely on old data without factoring in real-time updates.
» Bias in Data // Data sets used to train AI models are often not statistically significant. These issues can be as simple as dramatically better data being available in one neighborhood or from one provider, causing that data to overwhelm other, potentially more valuable data, in the AI’s analysis.
» Incomplete Data // I have never yet seen a property that is fully digitized. This is doubly the case for SFR properties, which are small in individual scale and highly varied. At best, the core characteristics are captured in the data, but there is always more missing than present in the digital record.
Without extensive, representative, timely, and high-quality data inputs, AI is always going to struggle. So as users of these tools, we need to make sure that we can feed it the right data if we want to be able to depend on the outcomes.
Missing Character, Intangibility, and Nuance
I was first attracted to real estate by its very “real” character. Unlike most financial assets, real estate is a living, breathing thing with character and life all its own.
This fact always hits home when I am touring properties, dating back to one of my first. I still remember that feeling of walking into a decrepit property in the northwest side of Chicago and seeing nothing but potential in the well-aged bones of an unusual property located in an edgy, but up-and-coming neighborhood.
That very potential — wrapped up in very human concepts like character — is extremely difficult to digitize and, as a result, remains beyond the reach of AI in this space.
Here are some examples of the most challenging gaps in understanding character for AI:
» Neighborhood Sentiment and Future Growth // AI can analyze current demographic and economic data, but it may struggle to capture the subtle, on-the-ground shifts that can indicate future neighborhood growth. Factors like new businesses, planned infrastructure projects, or changes in community dynamics are much more visible to humans through local knowledge and experience than through data.
» Property Condition and Renovation Quality // While AI can estimate the value of renovations or upgrades, it cannot fully evaluate the quality of craftsmanship, the durability of materials, or the aesthetic appeal of the property. Human judgment is crucial in evaluating whether improvements will attract residents or increase the property’s long-term value.
» Local Market Nuances // Some SFR markets have hyper-local characteristics that may not be fully captured by data. For example, two neighborhoods within the same city could have vastly different demand characteristics due to local attractions, schools, or even intangible qualities like “curb appeal.” AI models tend to overlook these nuances, relying instead on broad averages.
Over-Reliance on Historical Data
AI models often depend heavily on historical data to make predictions about future performance. This reliance can be problematic in several ways:
» Failure to Account for Disruptions // AI models may not be equipped to predict sudden changes in the real estate market, such as economic downturns, natural disasters, or major regulatory shifts. For example, during the COVID-19 pandemic, could AI models have predicted the spike in demand for suburban single-family rentals as people moved out of urban areas? Such “black swan” events are hard to predict based solely on past trends.
» Market Saturation Blind Spots // AI might predict continued growth in areas that are already saturated with rentals, potentially suggesting that investors buy in markets that may soon face an oversupply. Without forward-looking insights into construction projects, zoning changes, or future rental supply, AI could overvalue a hot market.
» Inability to Adapt to New Realities // Real estate markets are constantly evolving due to factors like technological advancements, demographic shifts, and changing work-from-home trends. AI models that are solely based on historical data may fail to adapt to new realities quickly, resulting in outdated predictions.
To be successful in using AI, understanding the age of the data it is using is critical. Knowing that there is a brand new BTR community arising around the corner from a scattered site opportunity could radically reshape an investment strategy. And it can be very hard to know whether the AI already knows about that type of new information.
Prediction is Hard
At their core, LLMs are predictive models. They are designed to predict what comes next, whether it is the next word in a sentence or the next data point in a set. But prediction is hard, especially in real estate—an industry literally shaping the future.
Real estate developers, owners and operators are, in fact, creating the future of the lived experience for all of us, making it notoriously tough for AI to predict outcomes. The vision of a capable developer is always going to see things that are not simply the logical next step in a process.
AI-driven tools predict resident behavior, property appreciation, and rental demand, but these are probabilities, not certainties. The reality is, real estate professionals constantly shift the very context in which AI is making its predictions, making our discipline particularly resistant to this kind of effort.
Where AI Can Help Us Most
With the right inputs and understanding of the tools, there is a clear path for all of us to enhance productivity and make better decisions with help from these tools.
Here are some things you could be doing now to take advantage of AI today:
» Speed up repetitive work // Every role in SFR has its share of repetitive, labor-intensive tasks, and there, AI can really help. Need to come up with unique descriptions for every property in a scattered site portfolio? AI can do that. Need to map a large portfolio that you are evaluating? Let the AI do it.
» Get the pricing right // Setting rents and sale prices has always been a challenge in our space, but newly available data sets from sources like Dwellsy, combined with AI, can help you get sharper on pricing than you have ever been. However, there is no fully automated solution. Great data, interpreted by AI, gives us a better chance at pricing things correctly — and will allow us to do so in a manner that is compliant with new regulatory constraints. But we cannot just leave it up to the machines if we want a successful outcome.
» Predictive maintenance // AI processes data from smart home devices and past maintenance records to predict when key systems — such as HVAC, plumbing, or electrical — might need repairs or replacement. This predictive capability reduces the risk of unexpected breakdowns and lowers maintenance costs over time. AI can also recommend optimal times for inspections or preventive maintenance, increasing property longevity and resident satisfaction.
» Portfolio monitoring // For investors, AI tools analyze market data, property performance, and economic trends to report on the performance of an existing property or portfolio. Then, it can help with the interpretation of the performance, helping draw attention to opportunities or sources of concern that might have been hiding in the data.
Human Oversight Will Always Be Necessary
While some may imagine (or live in fear of) AI being able to take on ever more of our day-to-day work, the limitations on the performance of AI in our space are severe and there is no clear path to addressing them at this time.
Perhaps the data set will eventually get granular enough or the understanding of human nature will get deep enough that we will be able to rely on AI for higher-order decision-making within SFR.
For the time being, that remains in the realm of science fiction. But, we can get more efficient and start to automate many of the least exciting and interesting aspects of our roles.